What is MLOps? Exploring Best Practices and Differences from DevOps
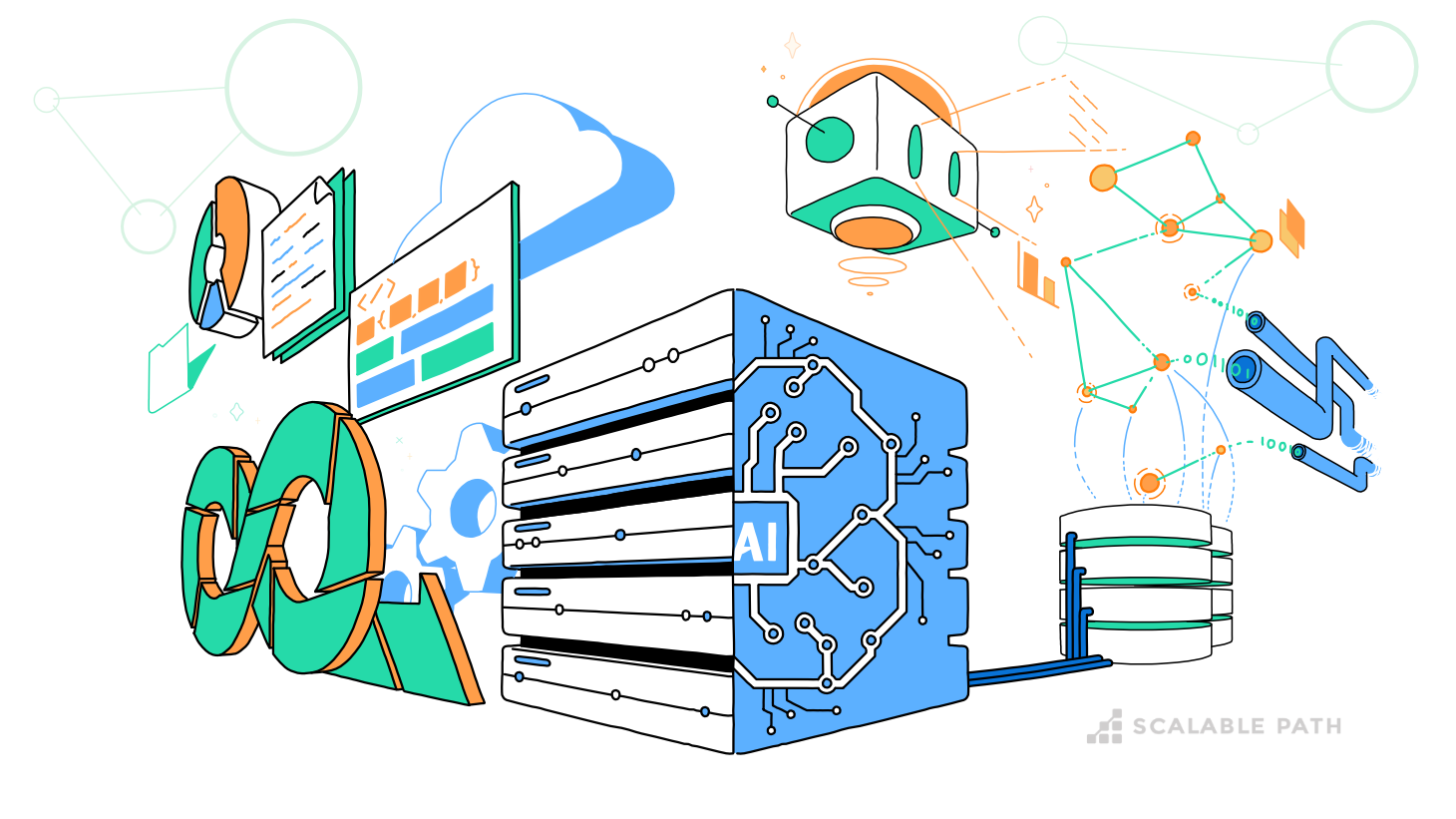
Machine learning (ML) has evolved from an experimental novelty to a fundamental part of the software landscape. As ML adoption has grown, development and deployment processes have had to adapt—and continue to evolve—to meet the demands of this field.
ML models are built at first for experimentation, and as such, typically lack robust infrastructure for large-scale testing and deployment. Instead, teams often rely on numerous manual processes, which lead to unique challenges as these models are transitioned to production environments. The main challenges include scalability, latency, model monitoring, and “model drift,” in which models lose accuracy over time as real-world data changes. These challenges highlight the need for robust systems that can adapt to evolving data and maintain performance in real-world applications.
Table Of Contents
In some ways, the software development industry has experienced this problem before – and addressed it – through the adoption of DevOps, which standardized and automated traditional software build pipelines. The application of DevOps to modern ML projects, however, uncovered the need for a more robust, ML-specific set of processes. As ML models transition from research projects to mission-critical systems, a new term has emerged: MLOps.
In this article, we’ll explore what MLOps is, why it’s so important, and how it differs from traditional DevOps practices.
Where it Started: The Introduction of DevOps
Before the introduction of DevOps, development and IT teams were often at odds with one another. While developers typically focus on rapid innovation and frequent code changes, IT teams want to keep systems secure and reliable. These disparate priorities often lead to information silos, inefficient deployment cycles, and frustration on both sides.
In an effort to bridge the gap between software developers and IT operations teams, Patrick Debois introduced the concept of DevOps in 2009. Through a set of business methodologies, tools, and practices, DevOps helps to facilitate a more collaborative and efficient software development lifecycle.
In case you’re a little rusty, here’s a brief refresher on the key principles of DevOps: