How to Hire a Machine Learning Engineer (Without Wasting Time or Money)
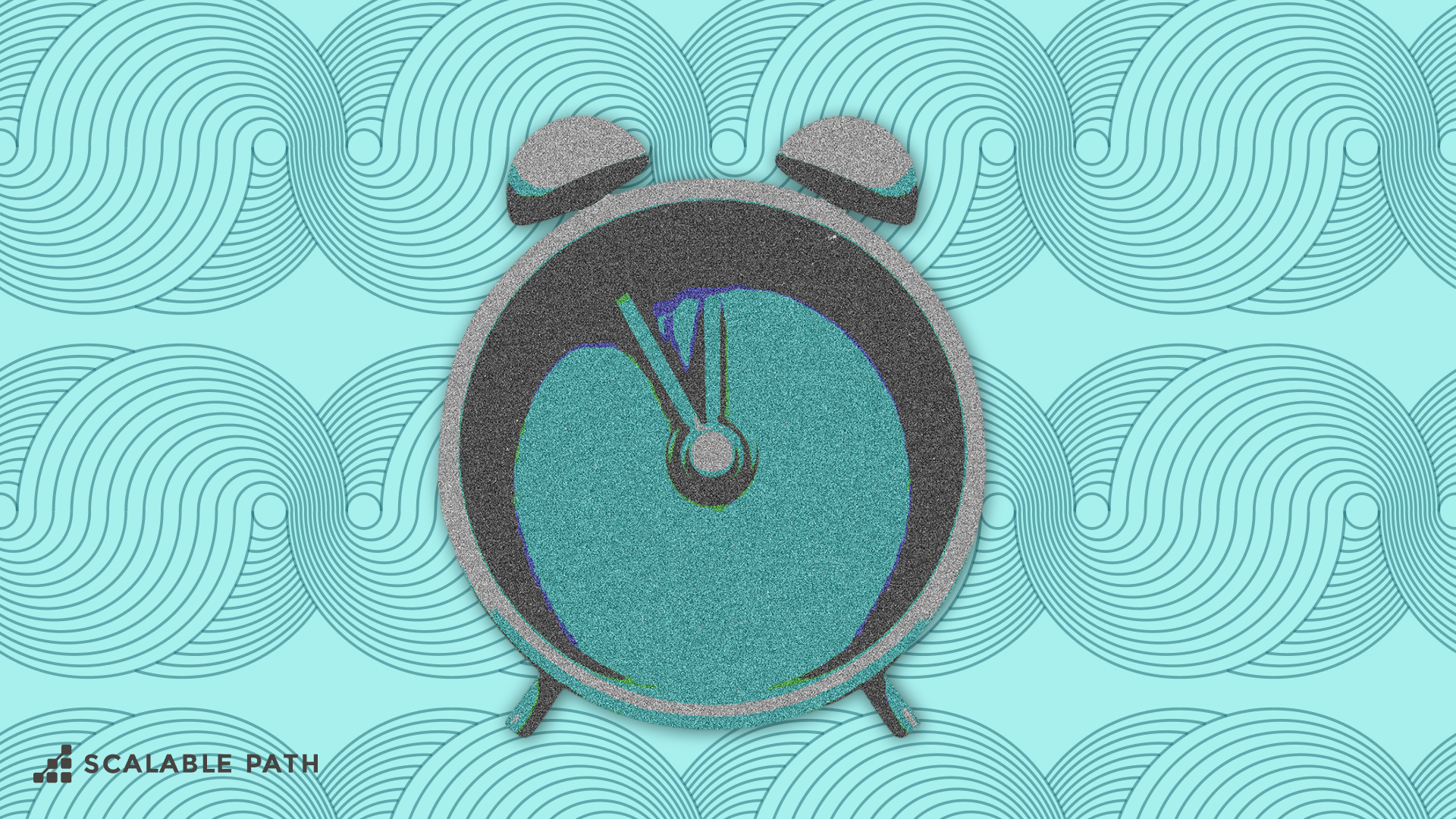
AI is evolving fast, but hiring the right talent to build with it? That’s a different story. Thanks to the rise of foundation models and the explosion of accessible tools—from open-source libraries to plug-and-play APIs—companies of all sizes are now experimenting with AI. However, turning those prototypes into real, scalable products takes a very different kind of team.
Table Of Contents
- Why Hiring Top Machine Learning Engineers Is a Whole Different Challenge
- Understanding the Role of a Machine Learning Engineer
- Data Scientist vs. Machine Learning Engineer
- Evaluating Candidates: Prioritize Real-World Experience Over Theory
- How Much Does It Cost to Hire a Machine Learning Engineer?
- How to Find the Right Machine Learning Engineer
- How to Write a Job Post That Actually Attracts ML Engineers
- Common Mistakes When Hiring an ML Engineer (and How to Avoid Them)
- Final Thoughts
The demand for machine learning engineers is expected to grow over 40% between 2023 and 2027. But there’s a problem: a lot of companies jump in without a clear plan for who they actually need. Do you need someone who can fine-tune models? Ship production code? Evaluate tradeoffs between off-the-shelf APIs and custom models? The answer depends on where you are in your AI journey—and it’s easy to get it wrong.
In this post, we’ll unpack what to consider before hiring a machine learning engineer: what skills actually matter, how to assess candidates (even if you’re not technical), what compensation looks like right now, and where the real challenges tend to show up.
Why Hiring Top Machine Learning Engineers Is a Whole Different Challenge
Hiring is almost always difficult, regardless of the role. But hiring a great Machine Learning Engineer is a different level of hard. There’s a few reasons for this.
First, the fundamentals are still evolving. AI and machine learning technologies are evolving fast; what was cutting-edge two years ago might be outdated today. Additionally, the pool of people who can actually build, deploy, and maintain real-world systems is small—and highly specialized.
It doesn’t help that many ML roles require years of hands-on experience just to reach competency, let alone mastery. And because these skills are in such high demand, top candidates are often already employed, earning competitive salaries and fielding multiple offers.
Still, that doesn’t mean hiring is hopeless. You just need to get clear on the type of ML talent you actually need—and where they’re likely to be. In the next section, we’ll break down how to define the role and set realistic expectations before you ever start sourcing.